Today, let's set cutting-edge machine learning and computer vision techniques aside. You probably already know that computer vision (or "machine vision") is the branch of computer science / artificial intelligence concerned with recognizing objects like cars, faces, and hand gestures in images. And you also probably know that Machine Learning algorithms are used to drive state-of-the-art computer vision systems. But what's missing is a birds-eye view of how to think about designing new learning-based systems. So instead of focusing on today's trendiest machine learning techniques, let's go all the way back to day 1 and build ourselves a strong foundation for thinking about machine learning and computer vision systems.
![]()
Allow me to introduce three fundamental dimensions which you can follow to obtain computer vision masterdom. The first dimension is mathematical, the second is verbal, and the third is intuitive.
On a personal level, most of my daily computer vision activities directly map onto these dimensions. When I'm at a coffee shop, I prefer the mathematical - pen and paper are my weapons of choice. When it's time to get ideas out of my head, there's nothing like a solid founder-founder face-to-face meeting, an occasional MIT visit to brainstorm with my scientist colleagues, or simply rubberducking (rubber duck debugging) with developers. And when it comes to engineering, interacting with a live learning system can help develop the intuition necessary to make a system more powerful, more efficient, and ultimately much more robust.
The illustration below depicts a linear classifier. In two dimensions, a linear classifier is a line which separates the positive examples from the negative examples. You should first master the 2D linear classifier, even though in most applications you'll need to explore a higher-dimensional feature space. My personal favorite learning algorithm is the linear support vector machine, or linear SVM. In a SVM, overly-confident data points do not influence the decision boundary. Or put in another way, learning with these confident points is like they aren't even there! This is a very useful property for large-scale learning problems where you can't fit all data into memory. You're going to want to master the linear SVM (and how it relates to Linear Discriminant Analysis, Linear Regression, and Logistic Regression) if you're going to pass one of my whiteboard data-science interviews.
Another great opportunity is to follow Adrian Rosebrock's pyimagesearch.com blog, where he focuses on python and computer vision applications.
Further reading: Old blog post: Why your vision lab needs a reading group
Homework assignment: First somebody on the street and teach them about machine learning.
NOTE: There a handful of other image recognition systems out there which you can turn into real-time vision systems, but be warned that optimization for real-time applications requires some non-trivial software engineering experience. We've put a lot of care into our system so that the detection scores are analogous to a linear SVM scoring strategy. Making the output of a non-trivial learning algorithm backwards-compatible with a linear SVM isn't always easy, but in my opinion, well-worth the effort.
Extra Credit: See comments below for some free VMX by vision.ai beta software licenses so you can train some detectors using our visual feedback interface and gain your own machine vision intuition.
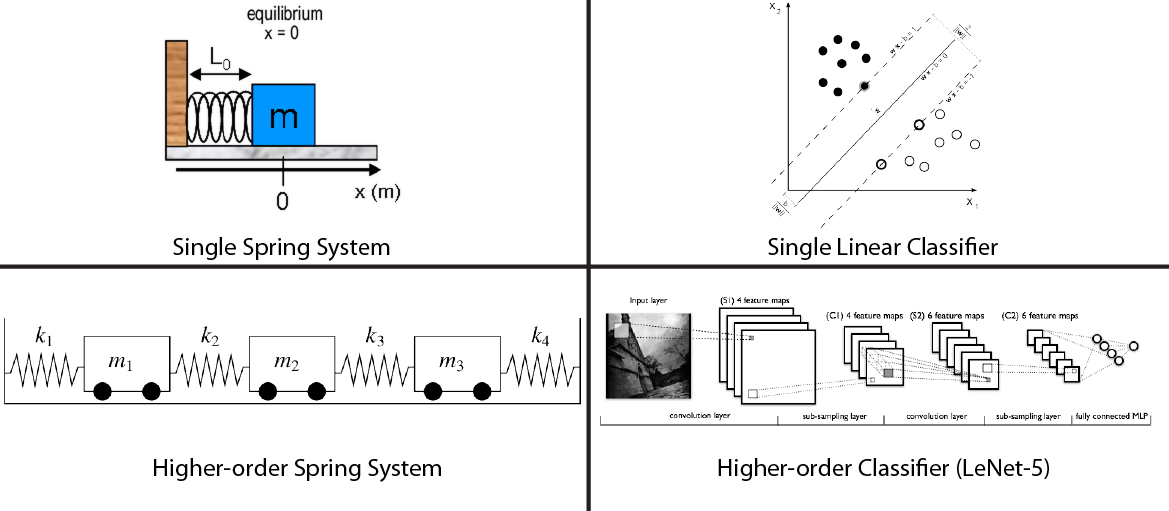
Allow me to introduce three fundamental dimensions which you can follow to obtain computer vision masterdom. The first dimension is mathematical, the second is verbal, and the third is intuitive.
On a personal level, most of my daily computer vision activities directly map onto these dimensions. When I'm at a coffee shop, I prefer the mathematical - pen and paper are my weapons of choice. When it's time to get ideas out of my head, there's nothing like a solid founder-founder face-to-face meeting, an occasional MIT visit to brainstorm with my scientist colleagues, or simply rubberducking (rubber duck debugging) with developers. And when it comes to engineering, interacting with a live learning system can help develop the intuition necessary to make a system more powerful, more efficient, and ultimately much more robust.
Mathematical: Learn to love the linear classifier
At the core of machine learning is mathematics, so you shouldn't be surprised that I include mathematical as one of the three fundamental dimensions of thinking about computer vision.
The single most important concept in all of machine learning which you should master is the idea of the classifier. For some of you, classification is a well-understood problem; however, too many students prematurely jump into more complex algorithms line randomized decision forests and multi-layer neural networks, without first grokking the power of the linear classifier. Plenty of data scientists will agree that the linear classifier is the most fundamental machine learning algorithm. In fact, when Peter Norvig, Director of Research at Google, was asked "Which AI field has surpassed your expectations and surprised you the most?" in his 2010 interview, he answered with "machine learning by linear separators."
The single most important concept in all of machine learning which you should master is the idea of the classifier. For some of you, classification is a well-understood problem; however, too many students prematurely jump into more complex algorithms line randomized decision forests and multi-layer neural networks, without first grokking the power of the linear classifier. Plenty of data scientists will agree that the linear classifier is the most fundamental machine learning algorithm. In fact, when Peter Norvig, Director of Research at Google, was asked "Which AI field has surpassed your expectations and surprised you the most?" in his 2010 interview, he answered with "machine learning by linear separators."
The illustration below depicts a linear classifier. In two dimensions, a linear classifier is a line which separates the positive examples from the negative examples. You should first master the 2D linear classifier, even though in most applications you'll need to explore a higher-dimensional feature space. My personal favorite learning algorithm is the linear support vector machine, or linear SVM. In a SVM, overly-confident data points do not influence the decision boundary. Or put in another way, learning with these confident points is like they aren't even there! This is a very useful property for large-scale learning problems where you can't fit all data into memory. You're going to want to master the linear SVM (and how it relates to Linear Discriminant Analysis, Linear Regression, and Logistic Regression) if you're going to pass one of my whiteboard data-science interviews.
Linear Support Vector Machine from the SVM Wikipedia page
An intimate understanding of the linear classifier is necessary to understand how deep learning systems work. The neurons inside a multi-layer neural network are little linear classifiers, and while the final decision boundary is non-linear, you should understand the underlying primitives very well. Loosely speaking, you can think of the linear classifier as a simple spring system and a more complex classifiers as a higher-order assembly of springs.
Also, there are going to be scenarios in your life as a data-scientist where a linear classifier should be the first machine learning algorithm you try. So don't be afraid to use some pen and paper, get into that hinge loss, and master the fundamentals.
Further reading: Google's Research Director talks about Machine Learning. Peter Norvig's Reddit AMA on YouTube from 2010.
Further reading: A demo for playing with linear classifiers in the browser. Linear classifier Javascript demo from Stanford's CS231n: Convolutional Neural Networks for Visual Recognition.
Further reading: My blog post: Deep Learning vs Machine Learning vs Pattern Recognition
Also, there are going to be scenarios in your life as a data-scientist where a linear classifier should be the first machine learning algorithm you try. So don't be afraid to use some pen and paper, get into that hinge loss, and master the fundamentals.
Further reading: Google's Research Director talks about Machine Learning. Peter Norvig's Reddit AMA on YouTube from 2010.
Further reading: A demo for playing with linear classifiers in the browser. Linear classifier Javascript demo from Stanford's CS231n: Convolutional Neural Networks for Visual Recognition.
Further reading: My blog post: Deep Learning vs Machine Learning vs Pattern Recognition
Verbal: Talk about you vision (and join a community)
As you start acquiring knowledge of machine learning concepts, the best way forward is to speak up. Learn something, then teach a friend. As counterintuitive as it sounds, when it comes down to machine learning mastery, human-human interaction is key. This is why getting a ML-heavy Masters or PhD degree is ultimately the best bet for those adamant about becoming pioneers in the field. Daily conversations are necessary to strengthen your ideas. See Raphael's "The School of Athens" for a depiction of what I think of as the ideal learning environment. I'm sure half of those guys were thinking about computer vision.
If you're not ready for a full-time graduate-level commitment to the field, consider a.) taking an advanced undergraduate course in vision/learning from your university, b.) a machine learning MOOC, or c.) taking part in a practical and application-focused online community/course focusing on computer vision.
The School of Athens by Raphael
An ideal ecosystem for collaboration and learning about computer vision
If you're not ready for a full-time graduate-level commitment to the field, consider a.) taking an advanced undergraduate course in vision/learning from your university, b.) a machine learning MOOC, or c.) taking part in a practical and application-focused online community/course focusing on computer vision.
During my 12-year academic stint, I made the observation that talking to your peers about computer vision and machine learning is more important that listening to teachers/supervisors/mentors. Of course, there's much value in having a great teacher, but don't be surprised if you get 100x more face-to-face time with your friends compared to student-teacher interactions. So if you take an online course like Coursera's Machine Learning MOOC, make sure to take it with friends. Pause the video and discuss. Go to dinner and discuss. Write some code and discuss. Rinse, lather, repeat.
Coursera's Machine Learning MOOC taught by Andrew Ng
Another great opportunity is to follow Adrian Rosebrock's pyimagesearch.com blog, where he focuses on python and computer vision applications.
Further reading: Old blog post: Why your vision lab needs a reading group
Homework assignment: First somebody on the street and teach them about machine learning.
Intuitive: Play with a real-time machine learning system
The third and final dimension is centered around intuition. Intuition is the ability to understand something immediately, without the need for conscious reasoning. The following guidelines are directed towards real-time object detection systems, but can also transfer over to other applications like learning-based attribution models for advertisements, high-frequency trading, as well as numerous tasks in robotics.
To gain some true insights about object detection, you should experience a real-time object detection system. There's something unique about seeing a machine learning system run in real-time, right in front of you. And when you get to control the input to the system, such as when using a webcam, you can learn a lot about how the algorithms work. For example, seeing the classification score go down as you occlude the object of interest, and seeing the detection box go away when the object goes out of view is fundamental to building intuition about what works and what elements of a system need to improve.
I see countless students tweaking an algorithm, applying it to a static large-scale dataset, and then waiting for the precision-recall curve to be generated. I understand that this is the hard and scientific way of doing things, but unless you've already spent a few years making friends with every pixel, you're unlikely to make a lasting contribution this way. And it's not very exciting -- you'll probably fall asleep at your desk.
Using a real-time feedback loop (see illustration below), you can learn about the patterns which are intrinsically difficult to classify, as well what environmental variations (lights, clutter, motion) affect your system the most. This is something which really cannot be done with a static dataset. So go ahead, mine some intuition and play.
Visual feedback is where our work at vision.ai truly stands out. Take a look at the following video, where we show a live example of training and playing with a detector based on vision.ai's VMX object recognition system.
To gain some true insights about object detection, you should experience a real-time object detection system. There's something unique about seeing a machine learning system run in real-time, right in front of you. And when you get to control the input to the system, such as when using a webcam, you can learn a lot about how the algorithms work. For example, seeing the classification score go down as you occlude the object of interest, and seeing the detection box go away when the object goes out of view is fundamental to building intuition about what works and what elements of a system need to improve.
I see countless students tweaking an algorithm, applying it to a static large-scale dataset, and then waiting for the precision-recall curve to be generated. I understand that this is the hard and scientific way of doing things, but unless you've already spent a few years making friends with every pixel, you're unlikely to make a lasting contribution this way. And it's not very exciting -- you'll probably fall asleep at your desk.
Using a real-time feedback loop (see illustration below), you can learn about the patterns which are intrinsically difficult to classify, as well what environmental variations (lights, clutter, motion) affect your system the most. This is something which really cannot be done with a static dataset. So go ahead, mine some intuition and play.
Visual Debugging: Designing the vision.ai real-time gesture-based controller in Fall 2013
Visual feedback is where our work at vision.ai truly stands out. Take a look at the following video, where we show a live example of training and playing with a detector based on vision.ai's VMX object recognition system.
NOTE: There a handful of other image recognition systems out there which you can turn into real-time vision systems, but be warned that optimization for real-time applications requires some non-trivial software engineering experience. We've put a lot of care into our system so that the detection scores are analogous to a linear SVM scoring strategy. Making the output of a non-trivial learning algorithm backwards-compatible with a linear SVM isn't always easy, but in my opinion, well-worth the effort.
Extra Credit: See comments below for some free VMX by vision.ai beta software licenses so you can train some detectors using our visual feedback interface and gain your own machine vision intuition.
Conclusion
The three dimensions, namely mathematical, verbal, and intuitive provide different ways for advancing your knowledge of machine learning and computer vision systems. So remember to love the linear classifier, talk to your friends, and use a real-time feedback loop when designing your machine learning system.